Accelerating COVID-19 Knowledge Synthesis w/ Machine Learning
Posted on Sunday, December 6th, 2020
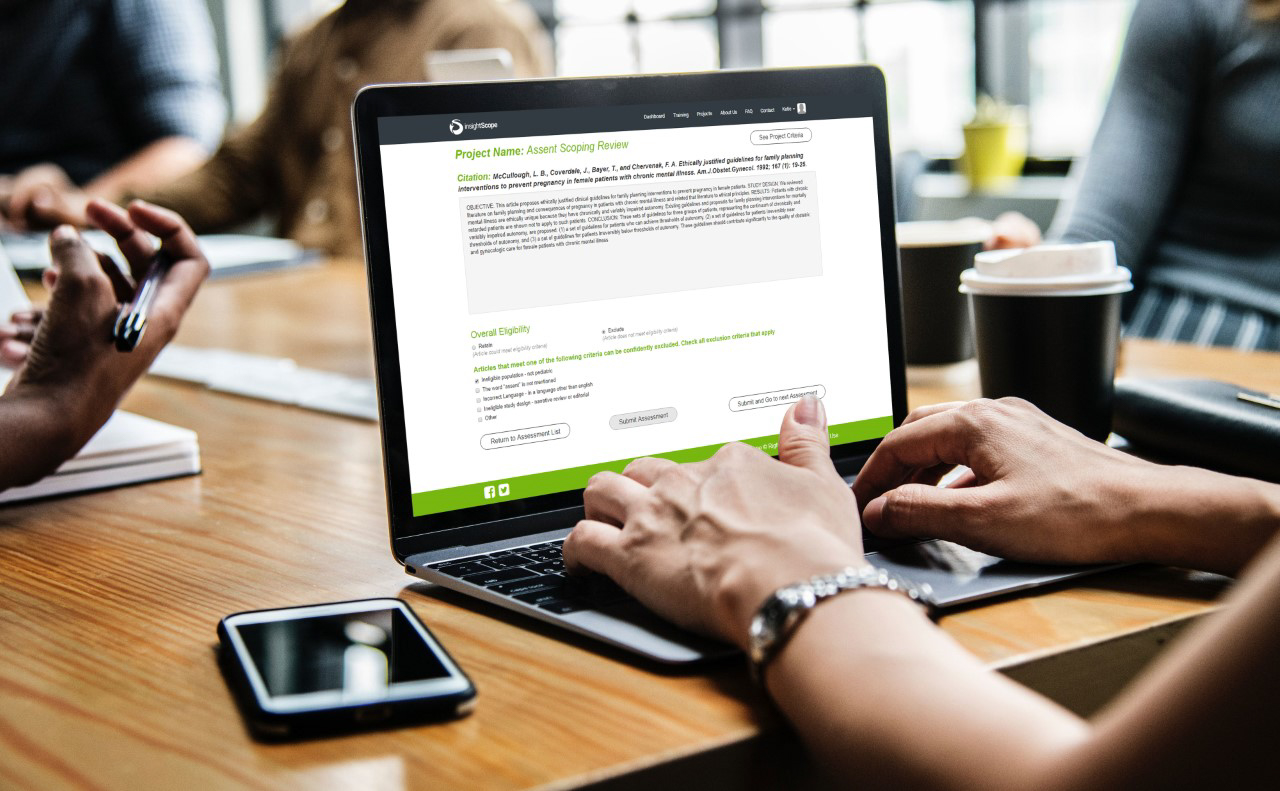
Client | insightScope |
Professor(s) | Kevin Holmes, |
Program | Computer Engineering Technology – Computing Science, Computer Programming, Mobile Application Design and Development |
Students | Slide Team: Ababiya Abajobir (Team Lead) (Student) (Computer Engineering Technology – Computing Science) Ryan Arreola (Student) (Computer Engineering Technology – Computing Science) Jaewoo Kim (Student) (Computer Programming) Ravi chandra Rachamalla (Student) (Mobile Applications Design and Development) Ilusha Rathnayake (Student) (Computer Programming) In collaboration with CHEO, insightScope and IBM Data Science & AI Elite Team and the IBM Machine Learning Hub Ottawa |
Project Description:
Responding to the COVID pandemic was challenging due to the lack of knowledge on many questions. Worldwide, clinicians and scientists rose to the occasion, rapidly initiating and completing a large research volume to address these questions. Comprehensively synthesizing the information in these studies, referred to as a systematic review, is challenging as it requires the assessment of hundreds or thousands of papers. The burden of completing these tasks usually falls on small teams of humans who must evaluate each article at least twice (independently). When completed by small groups, these reviews can take many months or years to complete, an unacceptable time frame when responding to a pandemic. Our client InsightScope created a platform that allows large teams (crowds) to work together to perform these tasks in a matter of weeks instead of months. Nonetheless, human resources are limited. It may be possible to complete more projects and more rapidly with artificial intelligence assistance.
This innovative project addresses the above challenge as follows:
First, we sought to evaluate whether that Machine Learning can achieve similar performance to humans in the identification of eligible studies across a range of systematic review with variable questions
Second, we sought to evaluate the extent to which incorporating a combined machine learning with a human approach to citation review reduces human assessors’ work.
We evaluated six different machine learning models, testing their performance on seven systematic reviews completed using crowdsourcing (large team), including those related to COVID-19. This process required us to review the platform database structure, develop the code, test, perform error analysis, interpret and make the necessary refinements. Through this process, we evaluated the machine learning predictions on over 100,000 human assessments. We used advanced technics such as stemming and lemmatization to improve artificial intelligence’s understanding of the publications. We were able to build an AI model that was able to identify 90% of the eligible articles on over 100,000 publications. This degree of accuracy is similar to what is known about the performance of a single human reviewer. After identifying the model with the best performance characteristics, we evaluated how replacing one of the human assessors with ML impacted study sensitivity and work saved.
When the machine assessment was considered along with the second assessment by a human, the combined retention of eligible papers was 98% combined. Our work demonstrates that it is possible to reduce the second human assessor’s job by at least 70% while retaining a high recall of eligible papers. This approach should make it easier to complete great knowledge synthesis efforts more rapidly while being highly comprehensive when adopted.
It has been a great learning and teaching opportunity. We’ve discovered Agile’s benefits, and using tools like Jira ensured the project moved along. We gained knowledge through communication and constant feedback that made both ourselves and the project better. We acquired the necessary skills through the documentation for AWS, Django, Python, Machine Learning, Git and much more. But above all, we learned that teamwork and collaboration get the job done!
Short Description:
With the world plagued by COVID-19, medical publications have swelled. Comprehensive reviews can take months or years. Machine Learning is the solution.
Gallery
![]() |
![]() |
![]() |
![]() |
![]() |
![]() |
Funded By